Is Snowflake The Right Solution for my Organization? We get asked this question so often that it prompted our team to write this post.
Founded in 2012, Snowflake Inc. the cloud-based data warehousing company, has so far transformed the traditional approach to data loading, storage, access and data governance in various industries. As a Snowflake partner, we get several enquiries about the Snowflake Data Cloud, its technology and suitability for different businesses. One of the most common queries we get is, whether Snowflake is good for their organizations.
So, is it time to upgrade from your legacy data on-premise or cloud platform to bring more agility to your business functions? Regardless if Snowflake is the right solution for your organization or not, updating and flexibilizing the data architecture is some of the most debated topics in today’s era of data-driven businesses.
Despite its numerous advantages, we understand Snowflake isn’t suitable for every business, so we thought of putting together the knowledge we acquired in the last few years to help you navigate the process of learning about and considering Snowflake for your organization’s environment. Let’s start from the basics before we delve deeper.
If you answer yes to at least one of the questions below, then we recommend you keep reading.
- Are you thinking of migrating on-premise databases to the cloud?
- Are you currently running Teradata, Hadoop or Exadata? Need to expand them without increasing overheads?
- Has compliant data-sharing now become paramount inside and outside your organization?
- Do you need to scale your database without impacting your production systems?
- Is your organization struggling with disparate sets of data?
What Is Snowflake?
Snowflake is a Cloud Data Platform delivered as a service. It’s a global ecosystem where customers, partners, and data providers can break down data silos and extract value from rapidly growing data sets in secure, governed, and compliant ways. It’s a centralized place where thousands of organizations have seamless and governed access to explore, share, and unlock the potential of their data.
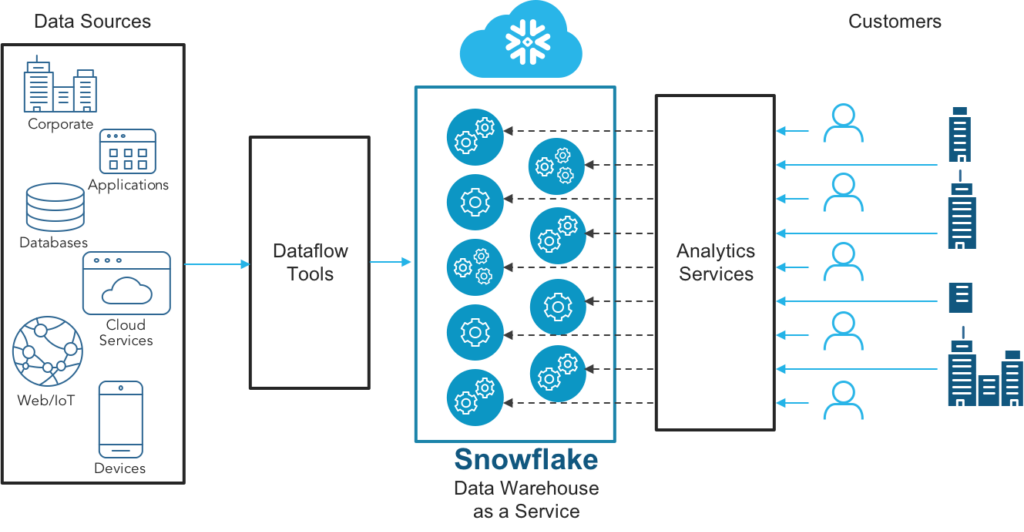
Snowflake architecture – Snowflake Docs
Why Snowflake?
So, what made Snowflake gain fast traction over the past decade? Apart from its unlimited advantages that we are going to discuss gradually, it is distinctive in how it responds to the shifting needs of enterprises. The Multi-Cluster Shared Data Architecture that Snowflake is based on allows it to provide a unified and seamless data experience. Snowflake’s cloud-based data warehouse as a service definitely stands out from the crowd.
On-premise data
Due to resource constraints with on-premise solutions, switching between various data projects can be time-consuming and ineffective. But many organizations are still conducting their data operations in this manner.
Frequently, engineers had to pause resource-intensive searches in order to scan a database for crucial customer information. The same data teams would regularly have to spread out the execution of queries over several nights to counteract the lack of compute resources.
However, businesses can now use their data to enhance the knowledge about their customers, products and their companies – without worrying about resource contention — thanks to a highly scalable, accessible, and affordable cloud data warehouse like Snowflake.
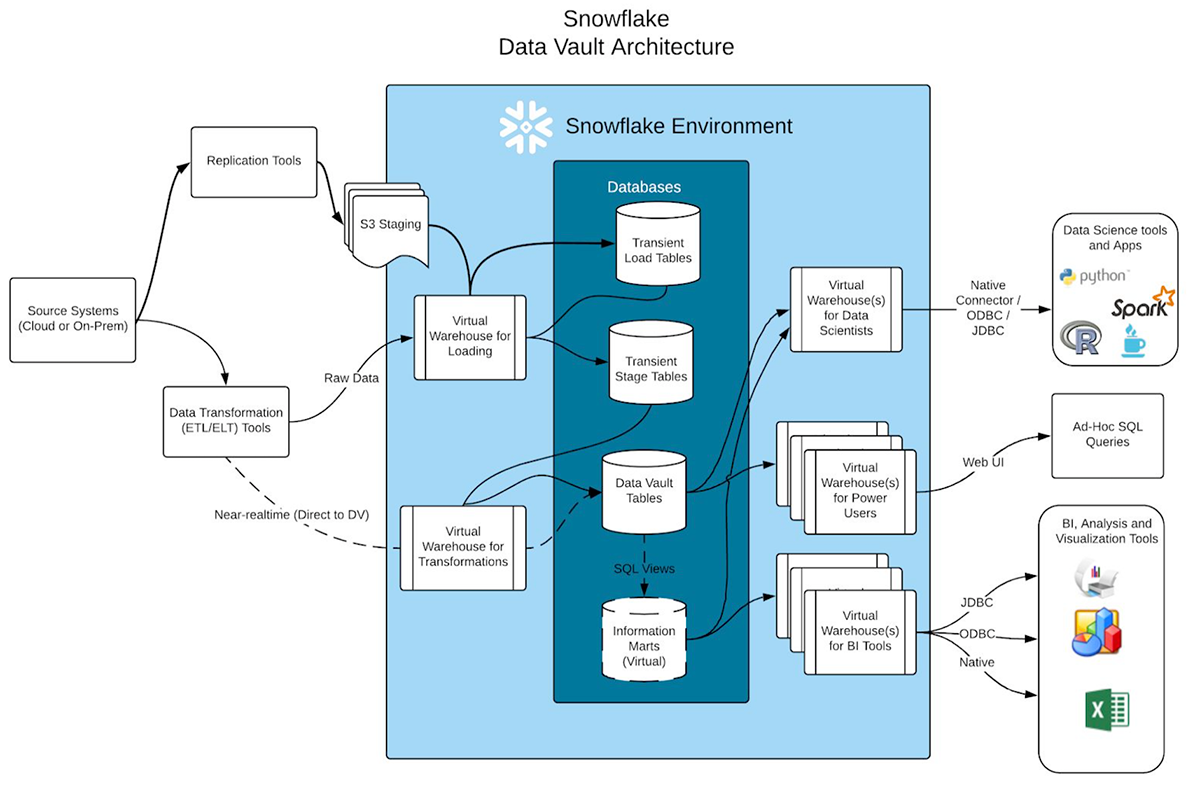
Snowflake Data Vault Architecture – Snowflake Docs
Snowflake can store and analyze all of your data records in one location. To load, integrate, and analyze data, it can automatically scale up/down by resizing a warehouse giving you faster queries results and also can scale out/in by adding clusters to a multi-cluster warehouse, allowing it to process multiple complex queries while avoiding contention.
Applications include batch data processing, streaming real-time data, providing interactive analytics, and processing complicated data pipelines. Take into consideration a common business situation where teams wish to execute multiple queries on client data to provide answers to various questions.
While your marketing team may be interested in learning about customer acquisition costs and customer lifetime value, your product team may be more interested in learning about engagement and retention.
So what distinguishes Snowflake from other data warehousing options?
The effectiveness and simplicity of use are the key factors. Analytics queries can be executed significantly quicker in Snowflake than they might in a conventional SQL (Structured Query Language) database because Snowflake has a micro-partitioning storage architecture that arranges data in columns. Let’s take a deep dive in the next section.
Limitations with On-Premise Databases
Scalability & Agility
Despite being the sole option available until recently, on-premise databases are physically hosted by a business’s own servers and behind a firewall, which puts constraints on organizations with a dispersed or globally distributed workforce. And they might still be able to meet your company’s demands. Cloud computing, on the other hand, has become very popular due to its improved, agile data delivery and easier architecture scalability (it can be resized as required by the business). And it can also prove to be cost-effective.
On-premise apps are dependable, secure, and give businesses control over their data that a cloud might lacks. However, IT decision-makers concur that they must use new cloud and SaaS apps in addition to their on-premise and legacy systems.
Data security will always come first, regardless of whether a business decides to maintain its apps on-premises or in the cloud. The choice of whether hosting their applications on premises may already be made for companies that operate in highly regulated sectors of the economy.
Limitations While Running Hadoop
Data Processing
MapReduce – essential to Hadoop – can only support batch processing. When processing a huge file, it requires the appropriate input and follows established instructions. The output from this method can prove very slow, which delays data processing and subsequent processes in managing and sharing your data.
Data Storage and Security
Because data security is included in Hadoop on numerous levels, your team must take particular care when keeping sensitive and important data. There is a strong probability of compromising these sensitive information is handled improperly.
Companies processing sensitive information must put in place the necessary security precautions. Your entire data set may be at risk since Hadoop eliminates protection mechanisms.
Hadoop employs native JavaScript language, like other frameworks do by default, which is a popular target for cybercriminals. Preventative measures are a must for data analysts using Hadoop.
Learning Curve
SQL is the language the majority of developers are used to, but Hadoop fully forgoes SQL in favor of Java. For those programmers and data analysts who want to use Hadoop, a thorough mastery of the Java language is required. They also need to be familiar with MapReduce in order to fully utilize Hadoop’s capabilities.
Limitations While Running Exadata
While the majority of developers prefer working with SQL and Hadoop focuses on Java, Oracle Exadata supports OLTP and OLAP database systems.
This brings several drawbacks, like the need to establish a portal to the free Oracle Platinum Support for Exadata. It isn’t always practical t do it in production systems containing critical data and requires complex patching on multiple layers. Also, any issue remedies require ongoing patching.
Your dynamic workload’s effectiveness may decline while running Teradata. Tactical workload is characterized by the primary index, so queries might only find one or a few rows.
To access one row, Teradata must transport 1MB from the disc to the FSG cache rather than 127.5 KB. This results in greater transfer costs and memory use. The same factor can also cause TPT Stream and TPump performance to decline.
The performance of row partitioned tables may suffer for two reasons: first, memory availability is mostly used in the sliding window merge join. Second, the NPPI table can only exist once a data block from each non-eliminated partition fits in the memory.
Regrettably, the larger data blocks require more memory, which will probably force Teradata to read the NPPI table multiple times. Joint efficiency will be reduced if a table is retrieved multiple times.
The danger will, as you might expect, be way greater for tables with numerous segments and few rows per division. Also, larger data blocks reduce the benefit of row splitting by increasing the likelihood that a data block contains rows from various divisions.
Challenges With Data Sharing
There can be a multitude of hurdles to data sharing for businesses with little or no experience with databases. Despite long, the list below doesn’t necessarily cover all challenges:
- Accidental release of private or sensitive information
- Delaying intentional release of information
- Concerns about your data being misinterpreted or exploited
- Improper data handling
- Compliance around sensitive data
- Policies that might restrict your access to larger data streams
- Approval procedures that are either too drawn-out or unclear
- Lack of understanding of the location of the data
- Confidentiality and security issues
- Insufficient resources making the data sharing project useless
- Presenting data in a visually appealing, shareable, and practical manner
- The violation of intellectual property rights and other interests
Data Compliance Issues
As data protection compliance requirements become more prevalent, CDOs regard data exchange as progressively problematic. This is especially noticeable when sharing data externally.
Numerous requirements demand a high level of transparency regarding the precise use of data, specific consent from subjects for data collection and use, and extensive auditing measures to demonstrate an agency’s adherence to all relevant laws and regulations. These stringent specifications may make restrict data sharing activities.
How to convert legalese into strategy is among the trickiest aspects of compliance in data sharing. The requirement for data use contracts between enterprises when information is shared externally adds to the complexity.
Lack of Proper Solutions & Security Issues
The use of advanced technologies by data engineering and operations teams to overcome some of the major obstacles to data sharing is not new for legacy databases and solutions. A lack of adequate tools and technology is a major problem with data sharing.
Technology is frequently seen as making data-related tasks simpler rather than more complex. However, a wide range of distinct cloud storage providers are now accessible, each providing a unique set of data access rules.
This disjointed mishmash of features frequently doesn’t expand reliably across many cloud data platforms, even if data teams continue to deploy several cloud data platforms at an accelerated rate. The end effects of extremely rigorous data policies is either completely prevent data sharing or overly broad policies that may allow for the leaking of sensitive data.
The outcome is either extremely rigorous data regulations that may completely prevent data exchange or overly lax policies that may allow sensitive data to fall through the cracks and result in a data breach or leak.
To get beyond this obstacle, you need a centralized, all-platforms data access control solution that separates policy from compute platforms, automates otherwise complex operations, like adaptive regulations and data audit reports, and works among all platforms.
Snowflake Architecture
The architecture of Snowflake is distinctive and avant-garde. Let’s have a quick glance at the Snowflake structure and its three layers.
Cloud Service Layer
It operates on compute clusters that Snowflake has deployed on various cloud providers and organizes Snowflake-wide operations.
Compute Layer
The computing layer is comparable to a virtual warehouse because it is made up of an infinite number of independently operating VIRTUAL WAREHOUSES.
Data Storage Layer
ALL data is directly and consistently saved using Data Storage Layer Storage Services (S3 on AWS, Blob Storage on Azure, or cloud storage on GCP). Snowflake stores all data in an efficient, specialized file format that is continually compressed and encrypted.
This program’s computing nodes all share a single disc or storage device. All processors have access to all drives even though each processing node (processor) has its own memory. Cluster control software is needed to monitor and regulate data analysis because all nodes have access to the same data. The data is uniformly copied across all nodes when it is changed or removed. The synchronous editing of the same data by two (or more) nodes must be prohibited.
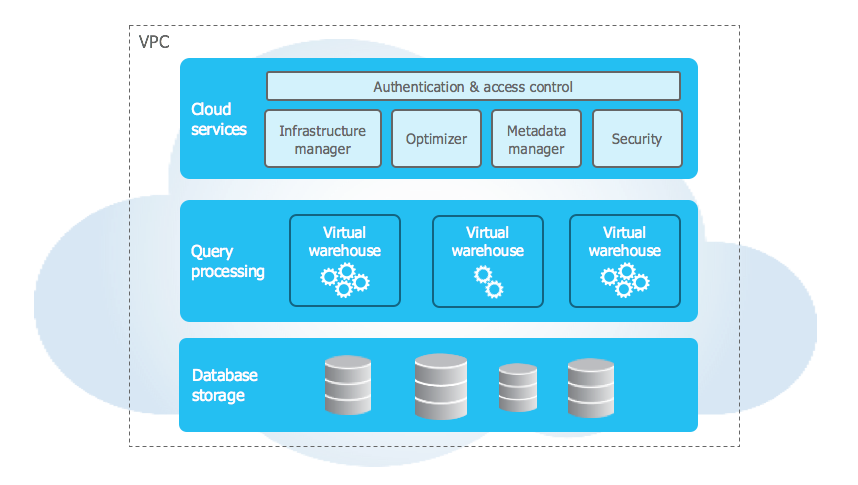
Source – Snowflake
Similar to other data warehouses, Snowflake needs its own query engine, natively supports structured and semi-structured data, and requires a proprietary data format. However, it is different from the conventional data warehouse in two important ways:
- It is only available in the cloud
- It separates the elastic compute layer from the storage
Teradata’s cloud Vantage service and Amazon’s Redshift RA (which separate storage from computation) have recently made an effort to replicate these features, but Snowflake’s innovation sets it apart.
Snowflake Architecture: How it Impacts Data Loading, Data Accessibility, and Data Governance
It’s important to choose a data platform that can handle large volumes of big data, fast speeds, and dependability, not to mention ease of use. Although the majority of players now offer cloud data platforms, many are assessing whether a data transfer may be necessary in order to remain competitive.
Snowflake, which functions as a cloud data warehouse and is popular for its capacity to support multi-cloud architecture setups, offers a simple solution for processing such data:
- Loading the data into a single column of type VARIANT,
- Addressing the data directly in data storage using additional tables, or
- Converting and loading the data into different columns in a typical relational table.
1. Data Loading in Snowflake
Snowflake enables businesses to load information from data warehouses, databases, backup data from security systems, sensors, chat logs, and Hadoop solutions in real time with minimal impact and in-flight transformations. With built-in scalability, security, and reliability, its architecture enables businesses to ingest information in real time from those on premise and cloud-based sources. By doing this, migration risks to Snowflake are reduced and operational decision-making has increased agility.
To load data directly from external sites, simply provide the location’s URL and your login information (given the location is secured).
The second step is managing a virtual warehouse. Each load will be performed by a warehouse, ingesting all data to the tables created into Snowflake databases.
The size of the warehouse affects load performance times.
Unstructured data includes photos, videos, and audio in addition to text-heavy content that is frequently found in form replies and social media interactions. This category includes file types that are specialized to certain industries, such as VCF (genomics), KDF (semiconductors), or HDF5 (aeronautics).
Snowflake promotes these behaviors:
- Securely access data files that are stored in the cloud
- Give partners and collaborators the URLs to your files
- Fill Snowflake tables with file access URLs and other file metadata
2. Data Governance in Snowflake
Snowflake derived many of the scalability capabilities that various cloud vendors provide and is accessible on AWS, Azure, and Google Cloud Platform.
In addition to the variety of cloud provider solutions, Snowflake adds the following features:
- Data node fault tolerance
- Automated data distribution among several data centers
- Time travel for data backups and retrieving deleted data
- Separating storage and computing resources
- Cloning and fail-over of data
For preserving data availability, these extra qualities are essential. For instance, if an AWS availability zone experiences a brief outage, Snowflake will handle multiple failure circumstances and divert your query off the inaccessible zone because your information and also your processing resources have been distributed across different availability zones.
Data Accessibility and Usability in Snowflake
When too many queries compete for resources in a typical data warehouse with a lot of users or use cases, you could incur in concurrency problems (e.g.: delays or errors). With its distinctive multi-cluster architecture, Snowflake handles concurrency challenges. Each virtual warehouse may scale up or down as needed, and queries from one virtual warehouse never affect queries from another. Without having to wait for other loading and processing processes to finish, data analysts and data scientists may acquire what they need when they need it.
It’s essential to set procedures and standards that ensure your data is accessible to users, properly recorded, identified, and labeled. Data ingestion must employ controls to provide reliable data. Services offer data in a variety of forms, so it’s essential that your tooling enforces the existing constraints set up by your business.
The centralized data approach used by Snowflake also encourages usability. A single data point may be referred to across different Snowflake accounts using its data sharing capabilities.
Snowflake also allows you to share data with reader accounts outside of your Snowflake instance taking the data accessibility to a whole new level.
Data Integrity in Snowflake
Your data must be dependable, accurate, whole, and consistent once it has been ingested and saved. To guarantee that your business can count on your data, you need set procedures in place. Physical and logical data integrity are the two main types that an organization using Snowflake ought to be mindful of. Let’s examine each of them individually.
It is essential that data can be reliably read and stored without data loss. The data should maintain its security in a duplicated context and when that availability zone or data center restores (in the event of the collapse of a data center or availability zone). In order to prevent data loss, Snowflake constantly duplicates your data from several availability zones.
In data governance, integrity means making sure that your data is logically correct and comprehensive in relation to your organization and particular domains.
To guarantee that you have the most recent data and that it adheres to the required types, values, and limitations, you must design and develop controllers within your data intake and storage.
You must establish controls and procedures apart from Snowflake in order to confirm the accuracy of the information. These controls will need assets outside of Snowflake to execute the comparison between your acquired data and the various locations it was imported from. The fact that Snowflake divides your read and write computation resources, however, means that transferring information out for verification has no effect on data ingestion.
3. Data Sharing in Snowflake
Secure Data Sharing allows you to share certain database items with other Snowflake accounts. Shareable Snowflake database objects include the following:
- Tables
- Outside tables
- Private views
- Firmly fabricated views
- Safe UDFs
No original data is duplicated or exchanged across accounts while using Secure Data Sharing. Snowflake’s special services layer and metadata storage enable all sharing. This is a key concept; it means that shared data does not utilize any storage space in a customer’s account and does not therefore contribute to the monthly data storage fees that the customer pays. Consumers only pay for the computing resources (such as virtual warehouses) used to query the shared data.
Additionally, Secure Data Sharing setup is quick and simple for providers and customer access to the shared data is immediate because no data is copied or exchanged. A read-only database is produced from the share on the user’s end. The same, common role-based access control that Snowflake offers for all system objects can be used to configure access to this database.
With the aid of this architecture, Snowflake makes it possible to establish a network of providers that can share data with numerous consumers (including those within your own company) and consumers that can access shared data from different sources.
To transform the data ingestion, storage, compliance and sharing for your business, talk to us.
- Zero-maintenance solution and seamless scaling
- Data-driven insights for improved operability and decision-making.
Get in touch with us to start today.